CNN: Specialized neural network for image tasks Learns spatial features used for object identification and picture categorization.
Numerous contemporary and cutting-edge AI applications leverage deep learning technology based on Convolutional Neural Networks (CNNs) to comprehend, interpret, and scrutinize diverse forms of video, audio, and textual data.
If you want to know about CNN start your informational journey with us.
Convolutional neural network español – Briefly Discuss!
A Convolutional Neural Network (CNN) is a specialized type of artificial neural network designed for tasks involving visual data, such as image and video analysis. It employs convolutional layers to systematically apply filters to input data, enabling the extraction of hierarchical features and patterns.
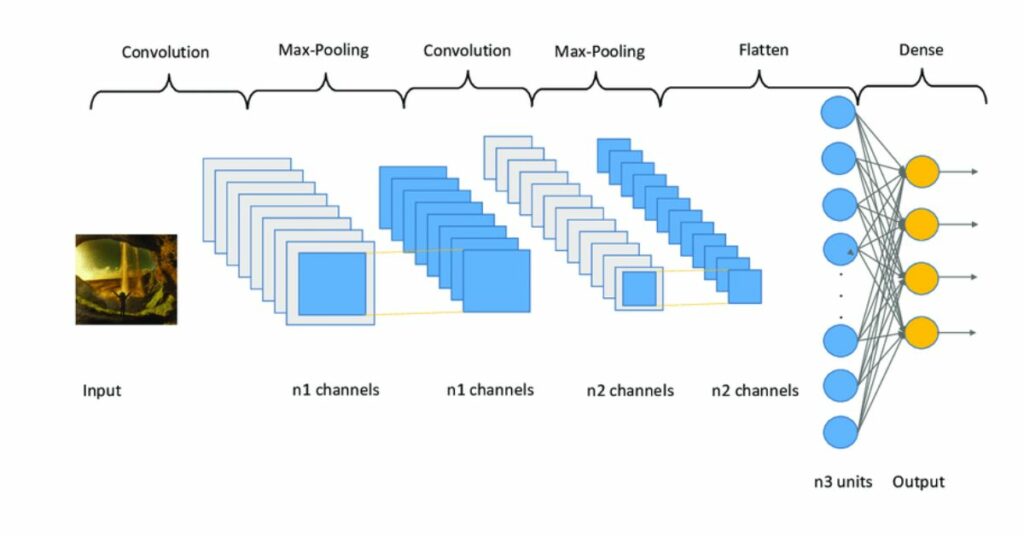
Key components include convolutional layers for feature extraction, pooling layers for spatial dimension reduction, and fully connected layers for complex decision-making. CNNs excel in image recognition, video analysis, and increasingly, natural language processing tasks.
They have become instrumental in fields like computer vision, autonomous vehicles, and medical imaging. Training techniques, such as data augmentation and transfer learning, enhance their performance.
Despite their successes, challenges like computational complexity and interpretability remain areas of active research as CNNs continue to evolve and find applications across diverse domains.
Key Components of Convolutional neural network español – Learn Something New Today!
Convolutional Layers:
CNNs employ convolutional layers to systematically apply filters to input data, enabling the extraction of spatial hierarchies and relevant features. This process facilitates the network’s ability to recognize patterns and objects.
Pooling Layers:
Pooling layers reduce the spatial dimensions of the input data, enhancing computational efficiency and reducing the risk of overfitting.
Fully Connected Layers:
Fully connected layers in CNNs contribute to the network’s capacity for complex decision-making. They connect every neuron from one layer to every neuron in the next, allowing the network to learn intricate relationships within the data.
Read Also: 2D Vector Images: The Growing Trend in Graphic Design
Applications of Convolutional neural network español – Let’s Explore!
Image Recognition:
CNNs excel in image recognition tasks, accurately identifying objects and patterns within images. This has significant applications in fields such as computer vision, autonomous vehicles, and medical imaging.
Video Analysis:
In the realm of video processing, CNNs play a crucial role in tasks like action recognition, object tracking, and scene understanding. Their hierarchical feature extraction makes them adept at handling temporal aspects of data.
Natural Language Processing (NLP):
While initially designed for visual data, CNNs have found utility in NLP tasks. They can effectively analyze sequential data, such as text, through techniques like text classification and sentiment analysis.
Autonomous Vehicles:
In the realm of autonomous vehicles, CNNs are instrumental for tasks like object detection, lane recognition, and obstacle avoidance. Their ability to process real-time visual data is crucial for ensuring the safety and efficiency of autonomous driving systems.
Artificial Intelligence in Gaming:
CNNs are widely used in the gaming industry for character recognition, scene understanding, and rendering realistic graphics. This enhances the gaming experience by creating more immersive and interactive virtual environments.
Read Also: 5 Best Reverse Image Search Tool – Detailed Guide In 2023
Training and Optimization of Convolutional neural network español – Let’s check!
- Training Process: CNNs are trained through backpropagation, adjusting parameters to minimize the difference between predicted and actual outputs.
- Data Augmentation: Techniques like rotation and scaling diversify the training data, enhancing generalization and preventing overfitting.
- Transfer Learning: Leveraging pre-trained models accelerates training and improves performance, especially with limited labeled data.
- Hyperparameter Tuning: Fine-tuning parameters like learning rates and batch sizes is crucial for optimizing CNN performance.
- Computational Efficiency: Addressing computational complexity involves exploring hardware optimizations and model quantization techniques.
Challenges and Future Directions – You may know!
Computational Complexity:
CNNs, particularly deep architectures, demand significant computational resources for training and inference. Addressing this challenge involves exploring hardware optimizations and efficient model architectures.
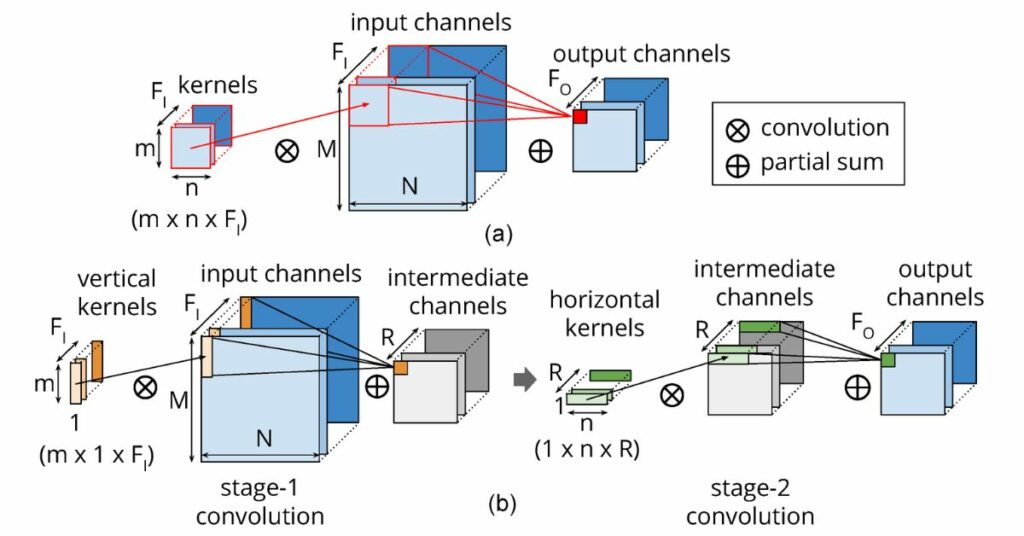
Interpretable AI:
As CNNs become more sophisticated, interpreting their decisions becomes a critical concern. Future research aims to enhance the transparency and interpretability of CNNs, especially in applications with high-stakes decision-making.
Robustness and Generalization:
Ensuring the robustness of CNNs to diverse real-world scenarios remains a challenge. Future efforts focus on improving generalization capabilities, addressing biases in training data, and enhancing the adaptability of CNNs across different environments.
Continual Learning:
Enabling CNNs to continually learn and adapt to evolving data distributions is crucial for applications in dynamic environments. Future research explores methods for continual learning to ensure that models remain effective over extended periods.
Read Also: The Benefits of Therapists Using HIPAA Compliant Emails
Deep Learning, Computer Vision, and CNN Applications Worldwide – Join the Community!
Overview of Deep Learning:
Deep Learning, a subset of machine learning, involves the use of artificial neural networks to simulate human-like decision-making processes. It has gained prominence for its ability to automatically learn and adapt from data, enabling the development of sophisticated models.
Significance of Computer Vision:
Computer Vision is a field within deep learning that focuses on enabling machines to interpret and understand visual information. It involves tasks such as image recognition, object detection, and facial recognition, with applications spanning from healthcare to autonomous vehicles.
The Role of Convolutional Neural Networks (CNNs):
Convolutional Neural Networks (CNNs) are a key architecture in deep learning, particularly tailored for tasks involving visual data. CNNs excel in feature extraction, making them instrumental in image and video analysis. Their hierarchical structure allows for the identification of complex patterns.
Applications in Healthcare:
Deep learning and computer vision find critical applications in healthcare, aiding in medical image analysis, disease diagnosis, and personalized treatment plans. CNNs, in particular, enhance the accuracy of detecting anomalies in medical images like X-rays and MRIs.
Autonomous Vehicles and CNNs:
CNNs play a pivotal role in the development of autonomous vehicles. They are used for object detection, lane recognition, and obstacle avoidance, contributing to the safety and efficiency of self-driving cars.
Retail and Visual Recognition:
In the retail sector, deep learning and computer vision are utilized for visual recognition tasks. This includes inventory management, cashier-less checkout systems, and personalized shopping experiences through facial recognition.
Read Also: What Is Included in a Brand Design? Check In 2023
Security and Facial Recognition:
Facial recognition powered by deep learning algorithms is employed in security systems worldwide. It is utilized for access control, surveillance, and identity verification in various contexts, from airports to smartphones.
Manufacturing and Quality Control:
Deep learning technologies, especially computer vision, enhance quality control in manufacturing. CNNs are used to inspect products for defects, ensuring high precision and efficiency in production processes.
Agriculture and Precision Farming:
Computer vision is revolutionizing agriculture with applications in precision farming. Drones equipped with deep learning algorithms can analyze crop health, optimize irrigation, and monitor overall farm conditions.
Global Impact and Future Trends:
The global impact of deep learning, computer vision, and CNN applications continues to expand across industries.
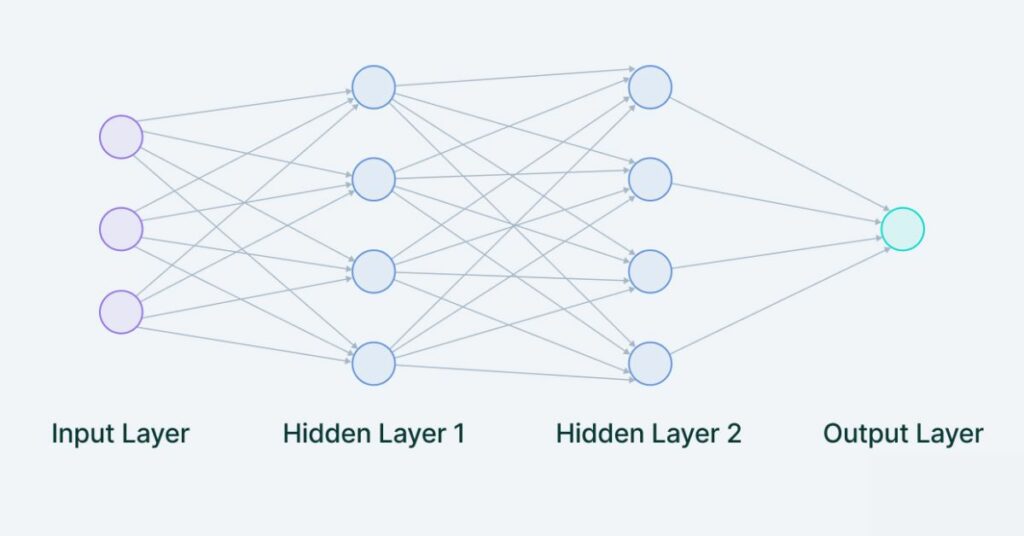
Future trends include advancements in interpretability, addressing ethical considerations, and the integration of these technologies into everyday life, shaping the next generation of intelligent systems.
Frequently Asked Questions:
1. How are Convolutional neural network español trained?
CNNs are trained using labeled datasets, adjusting parameters through backpropagation to minimize prediction errors.
2. What challenges do Convolutional neural network español face?
Challenges include computational complexity, interpretability, and addressing biases in training data.
3. What is the future of Convolutional neural network español research?
Future research focuses on overcoming challenges, enhancing interpretability, and exploring ethical considerations to expand CNN applications.
Conclusion:
At the end of the conclusion;
CNNs are a specialized type of neural network designed for image-related tasks, focusing on learning spatial features. They are employed for tasks such as identifying objects and categorizing pictures.
I hope you will understand all the concepts of Convolutional neural network español.